Tianyang Liu
Ph.D. Student at UC San Diego
til040 🌀 ucsd ✨ edu Click to copy
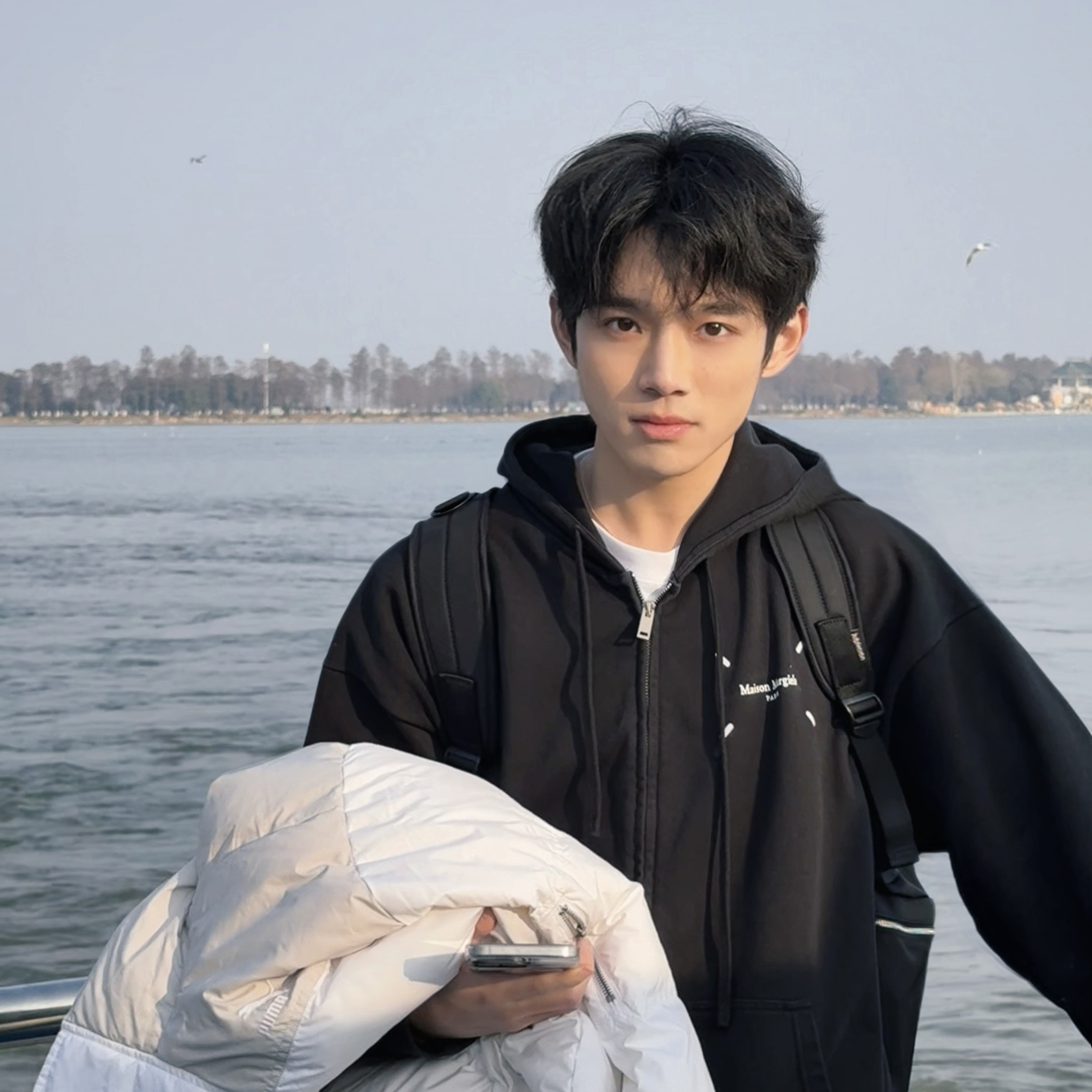
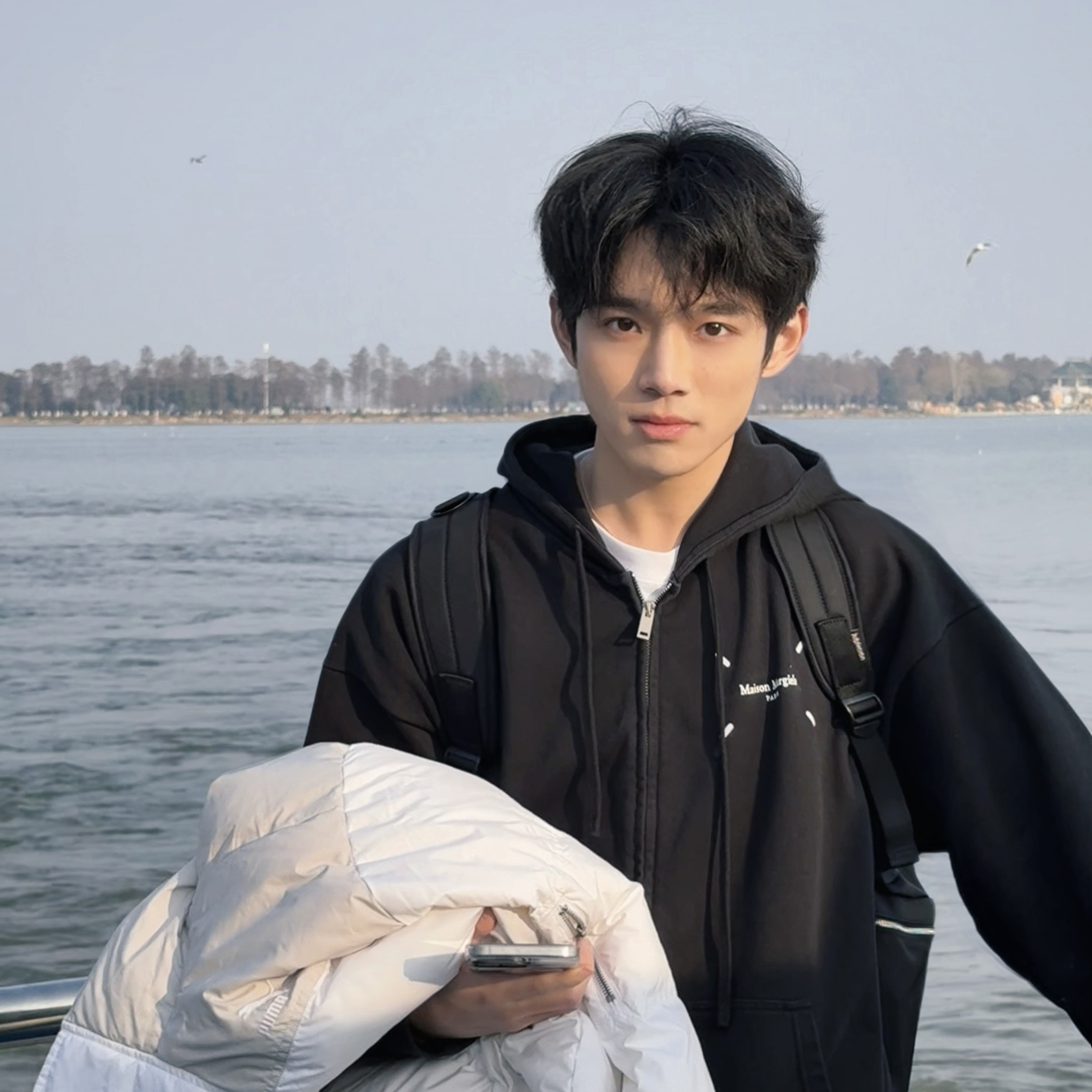
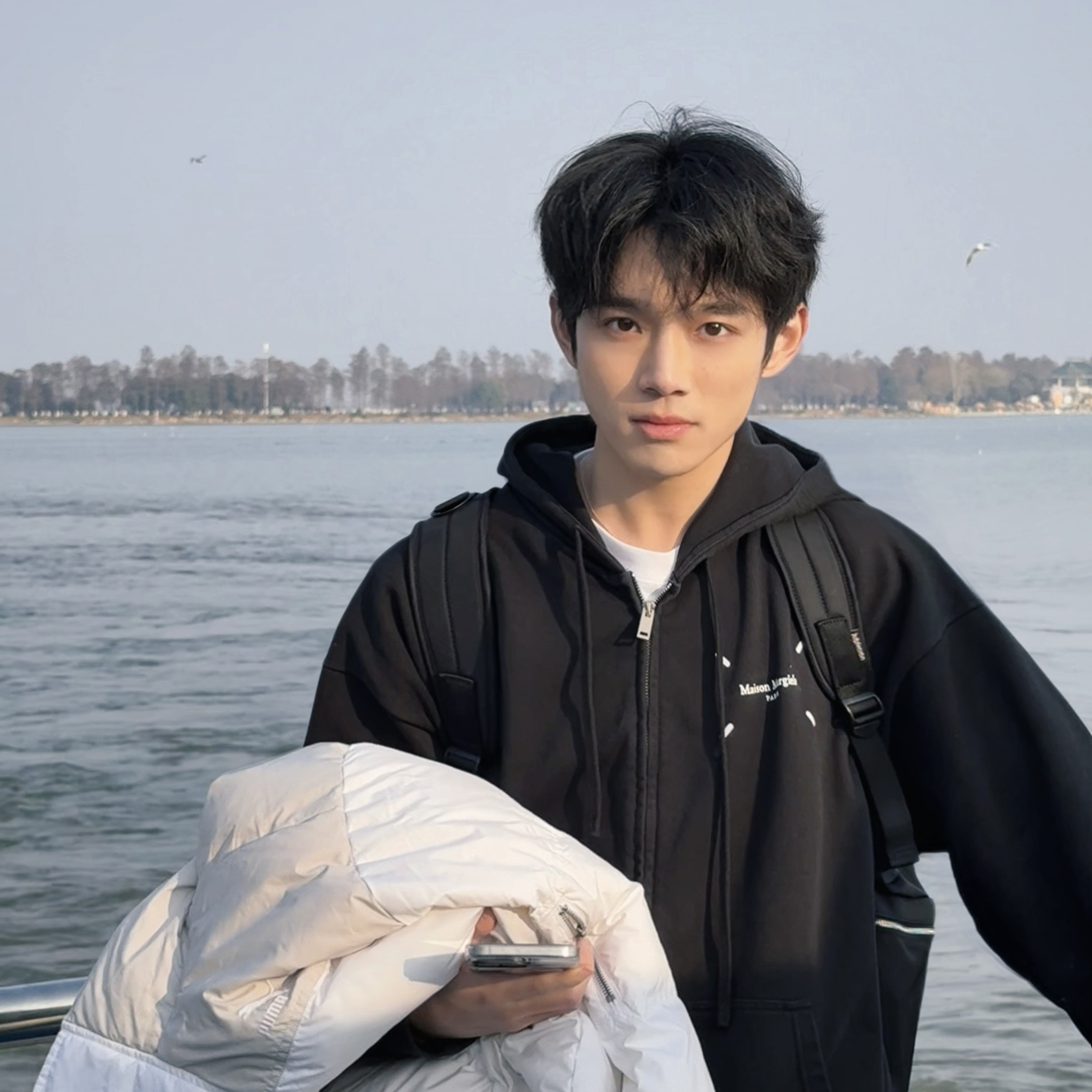
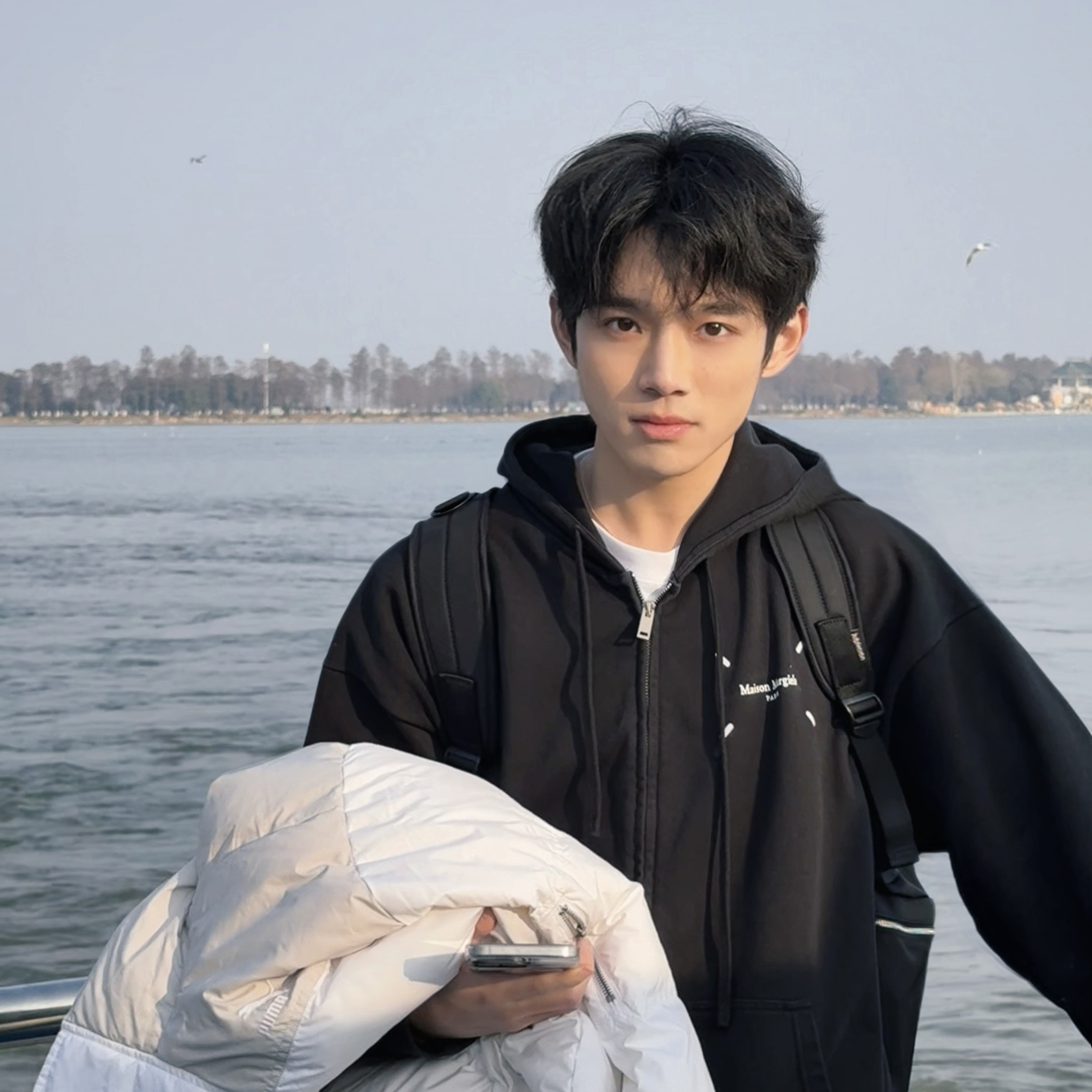
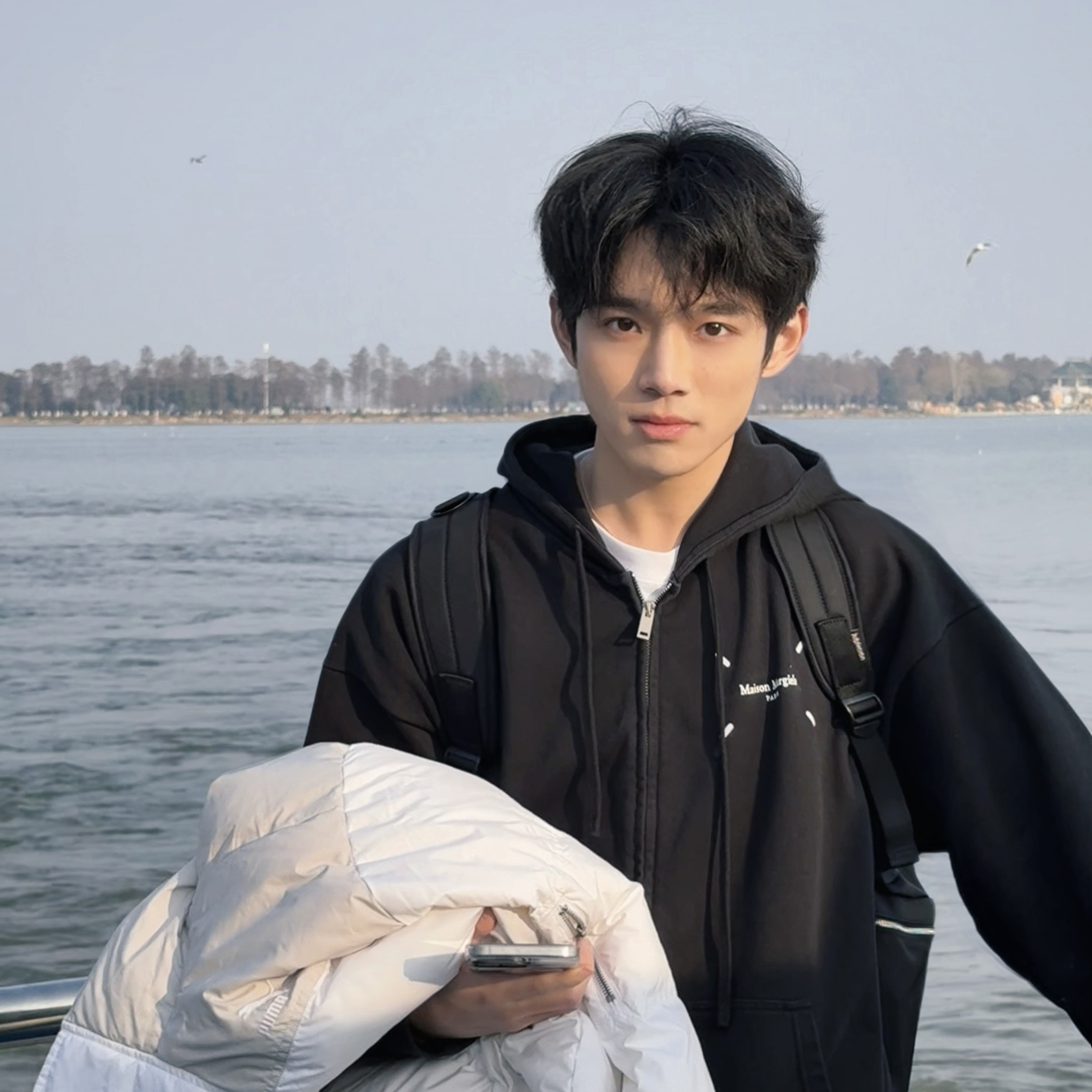
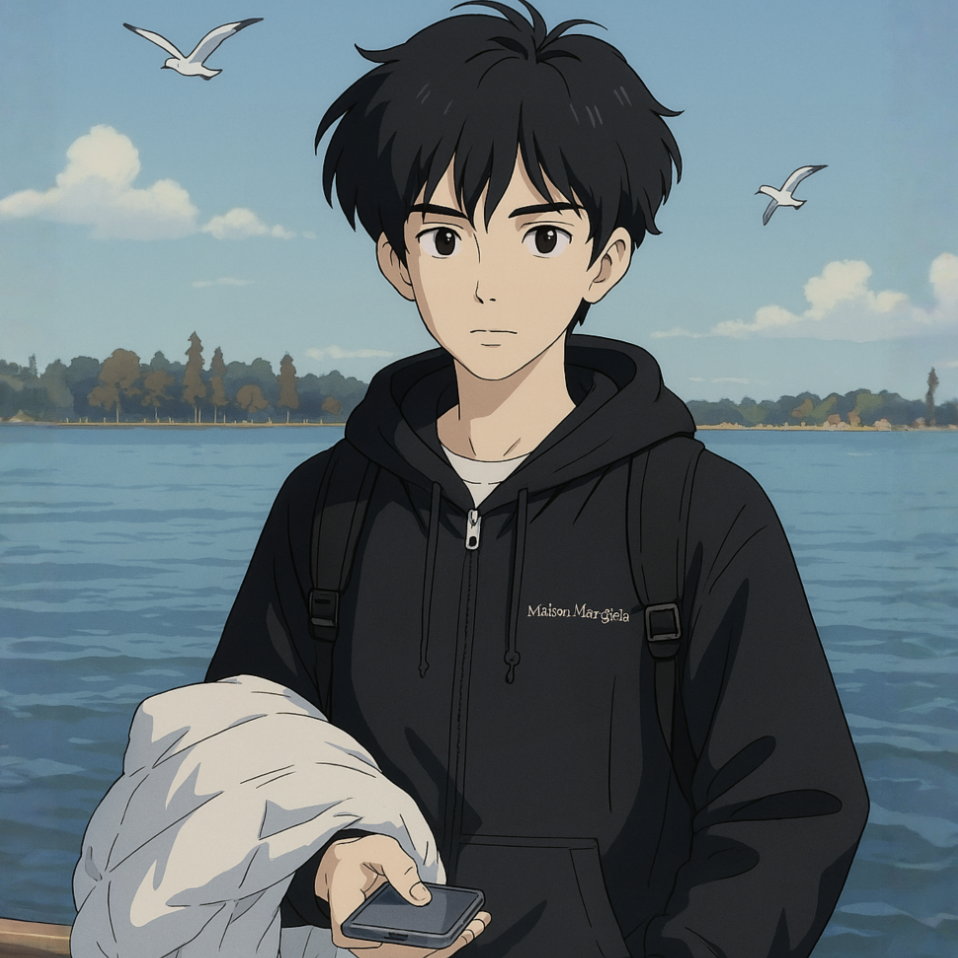
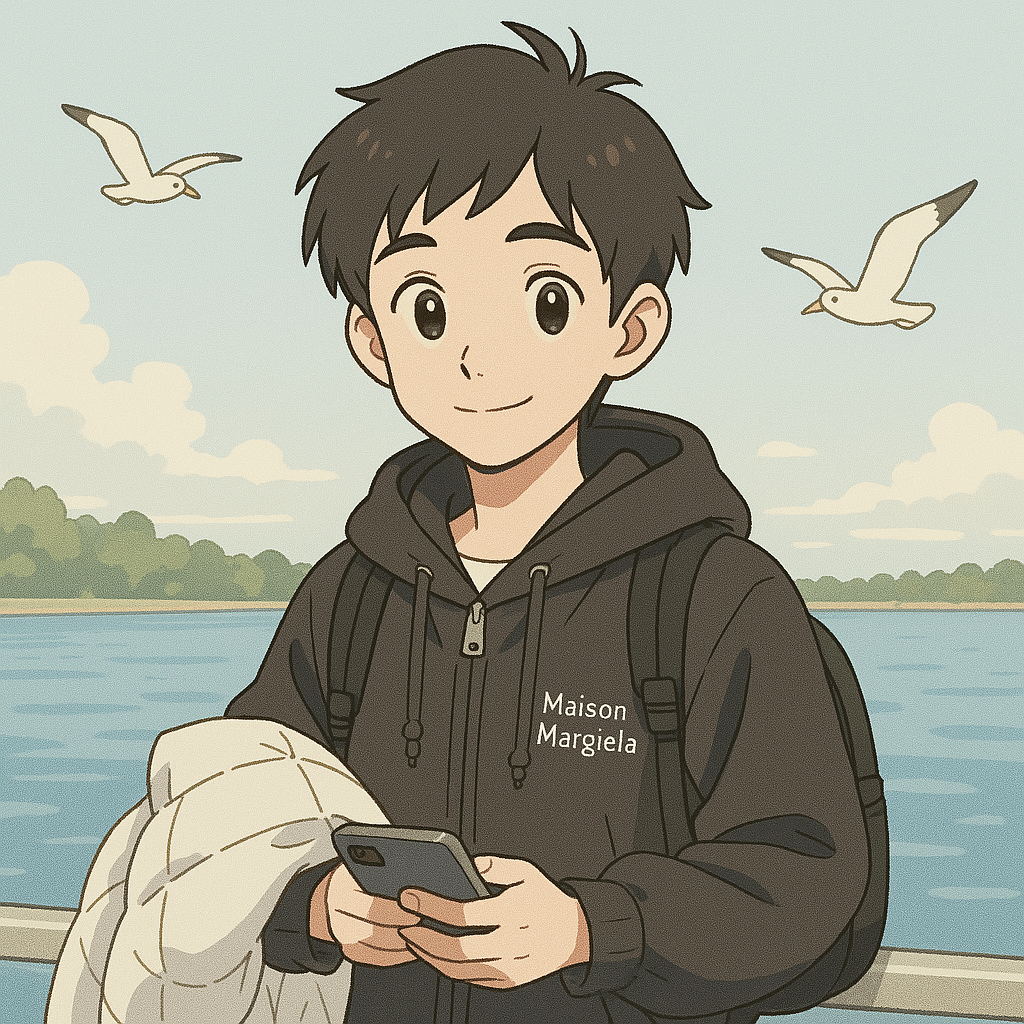
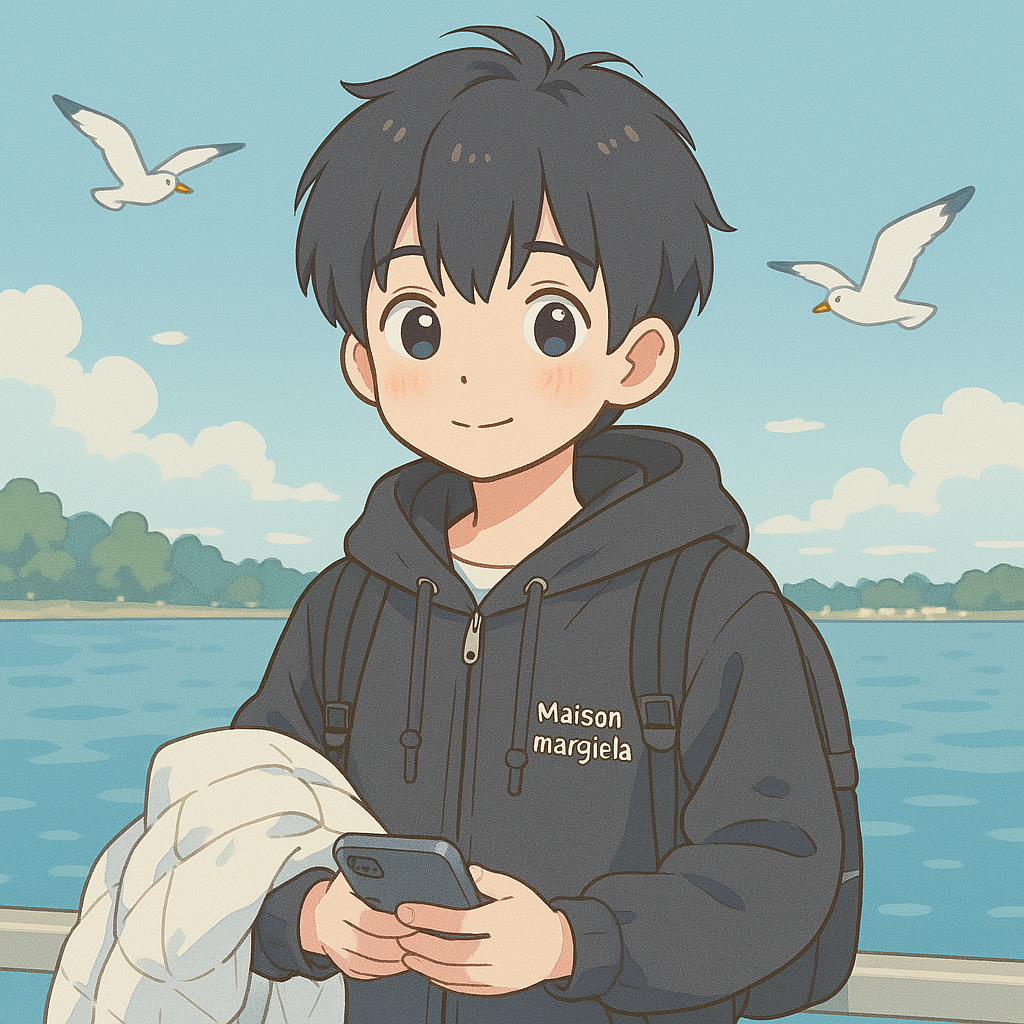
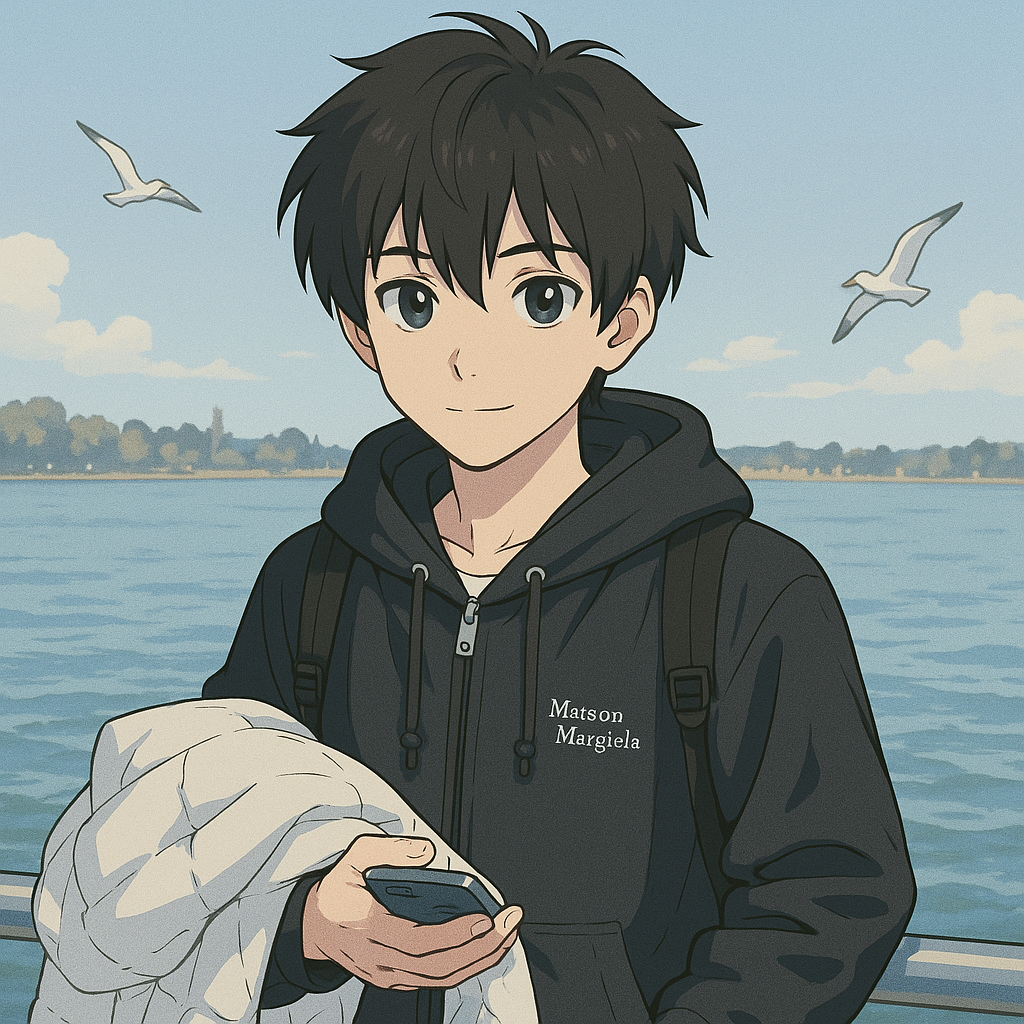
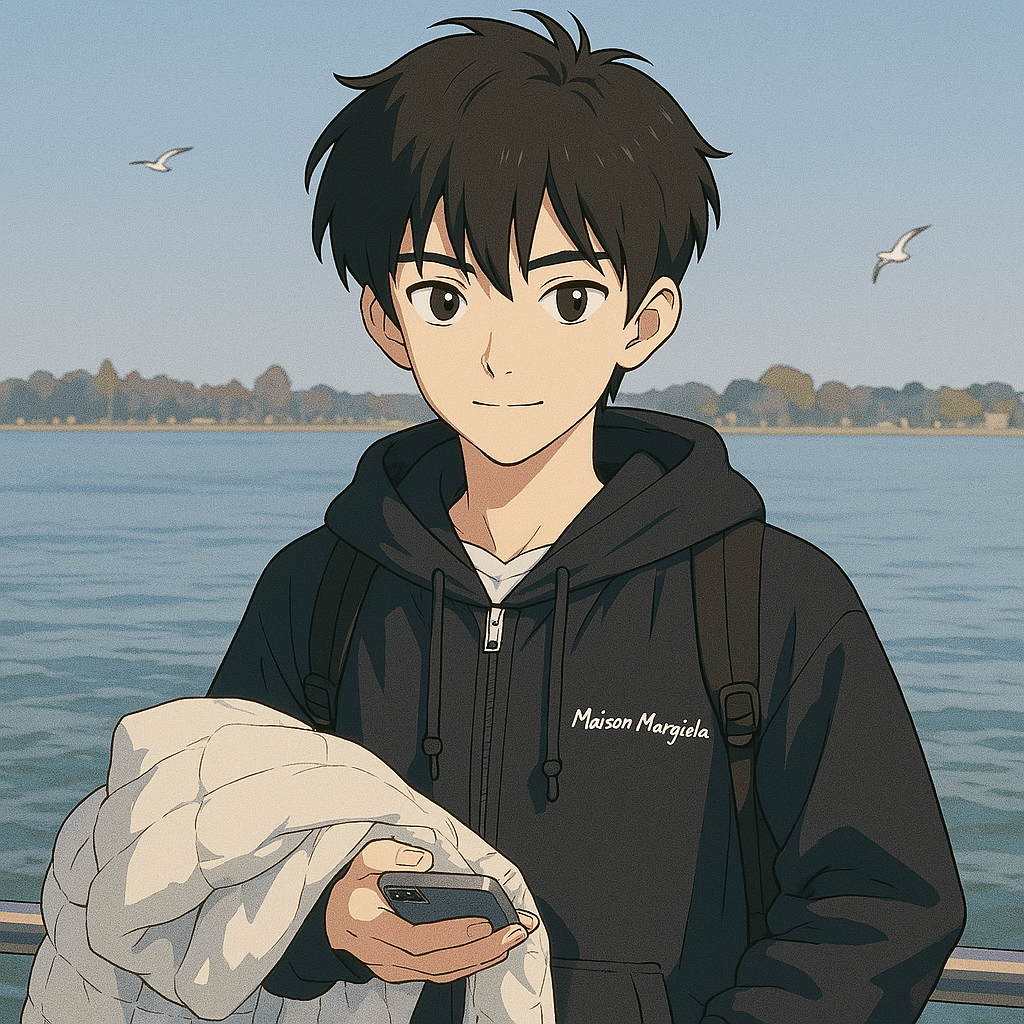
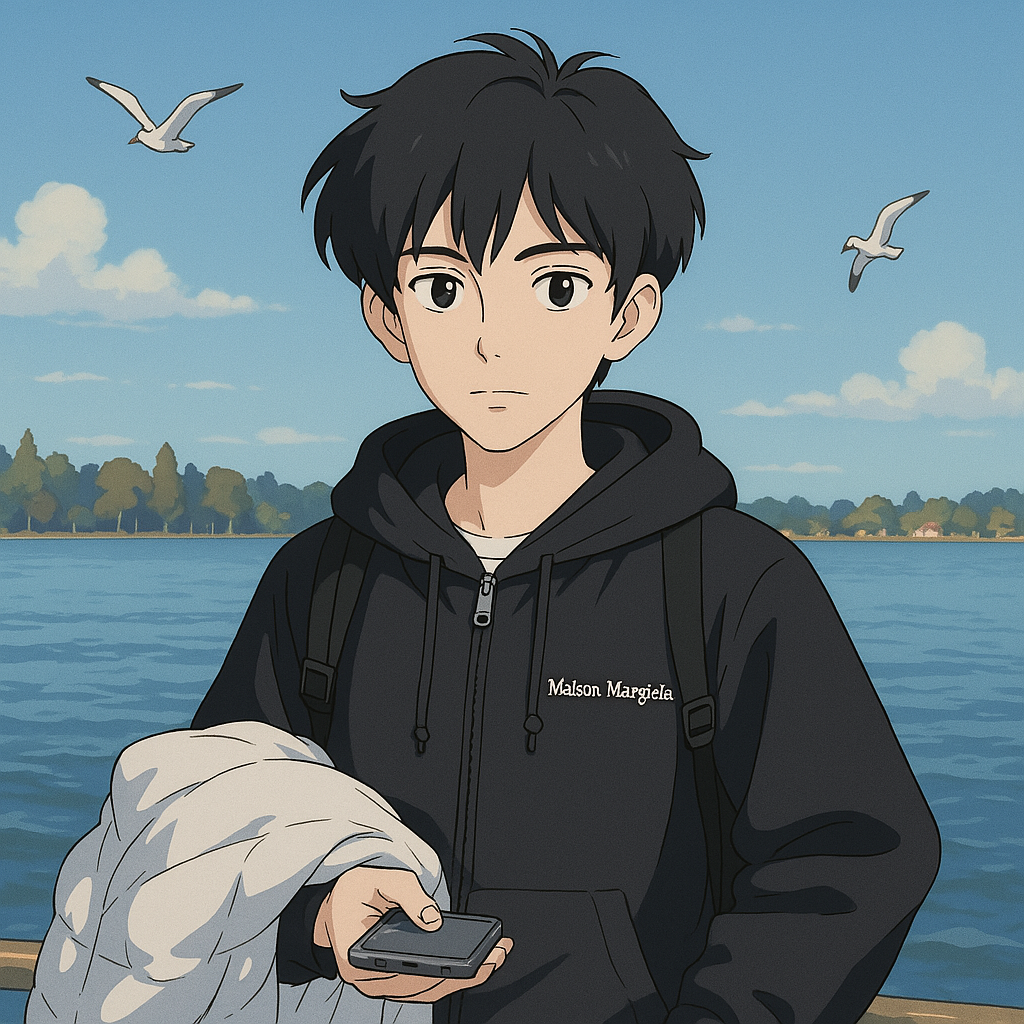
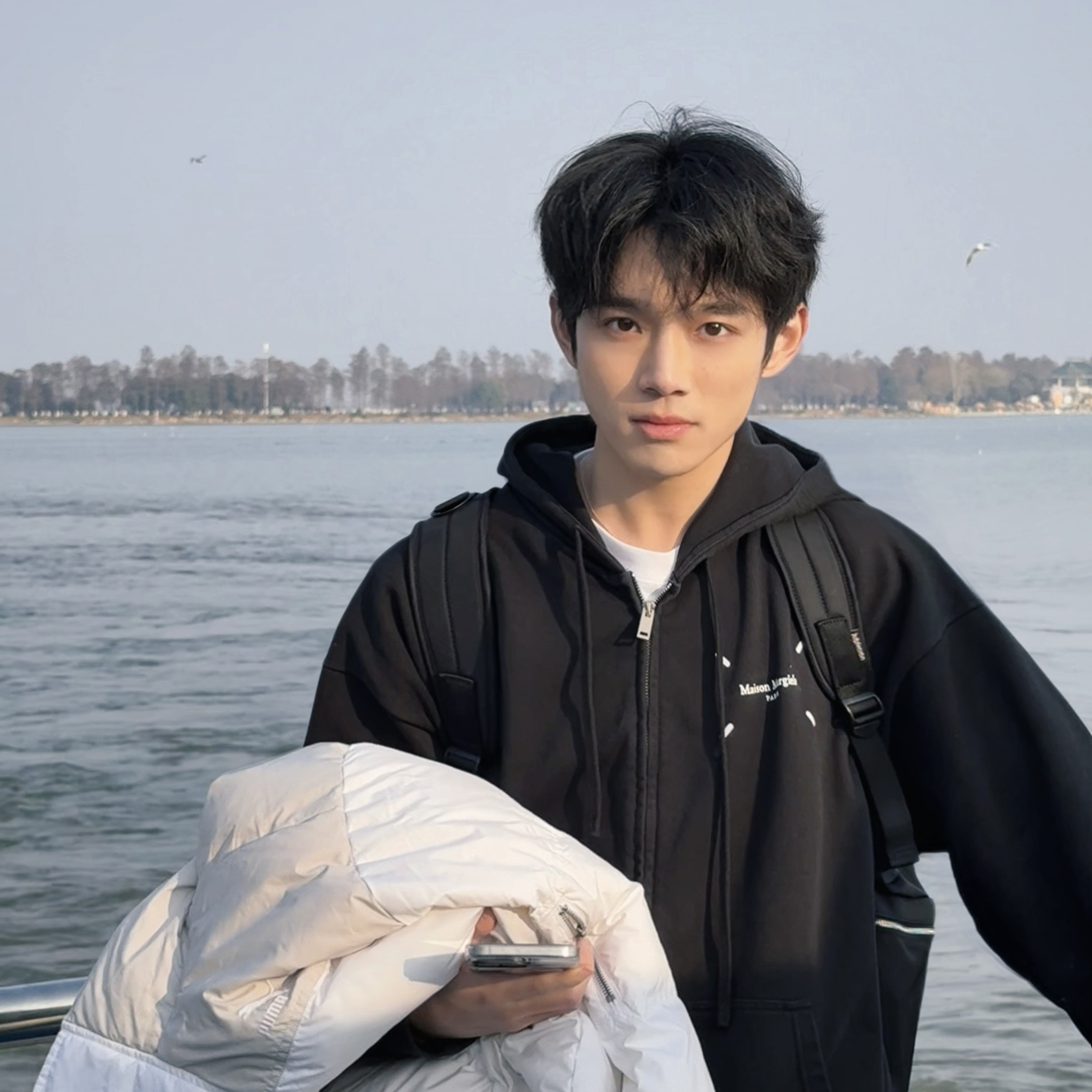
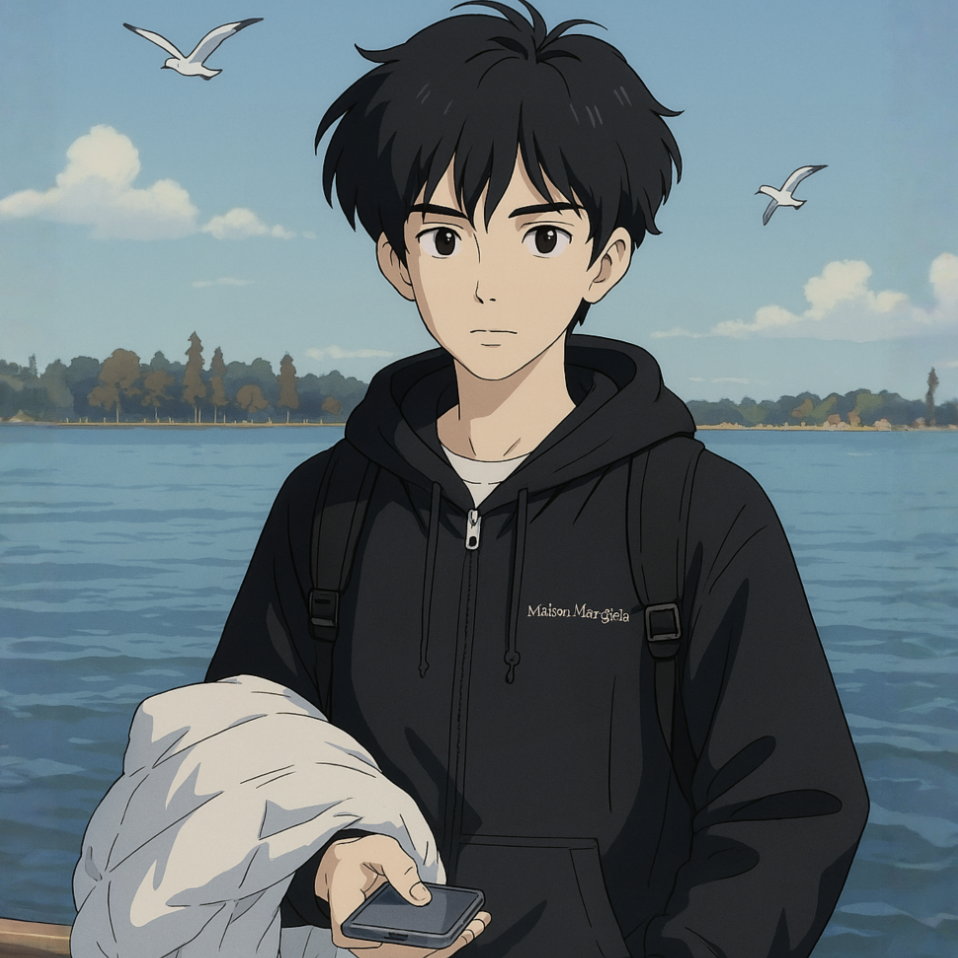
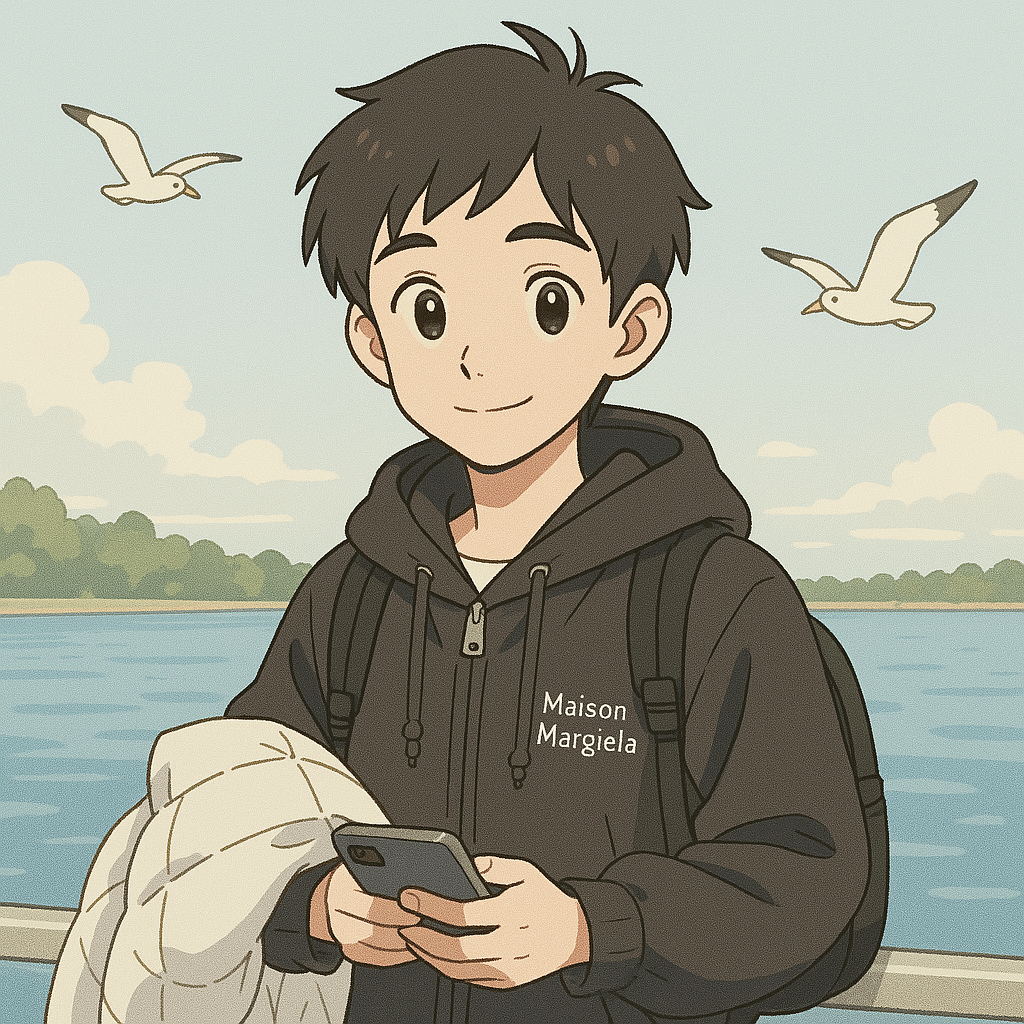
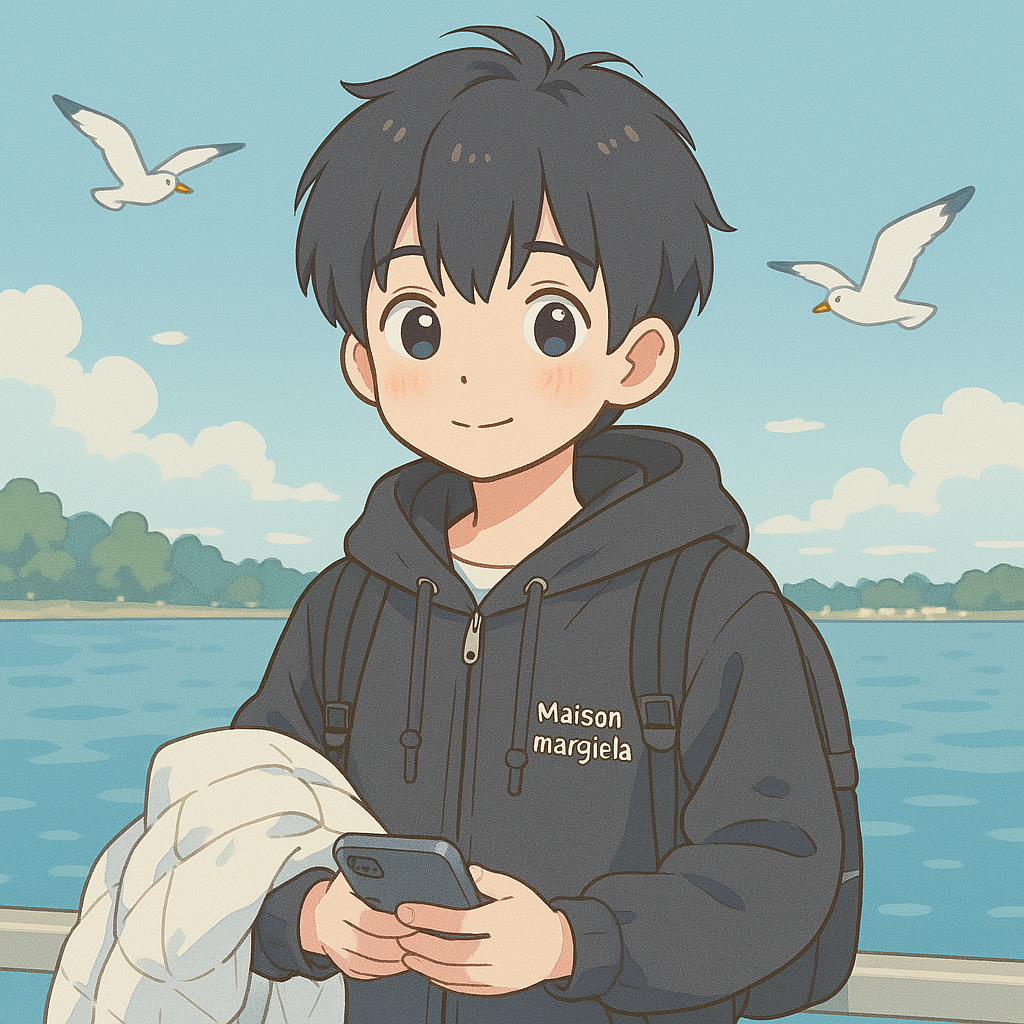
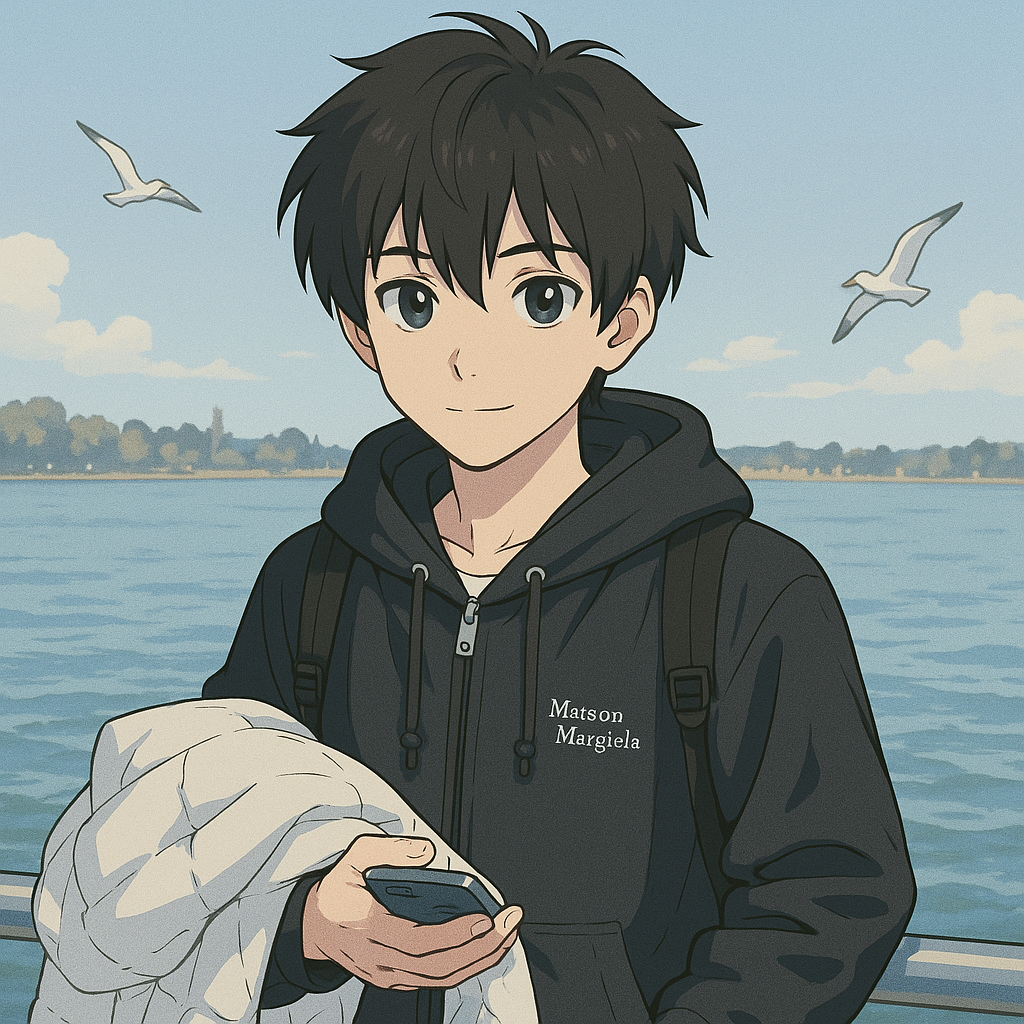
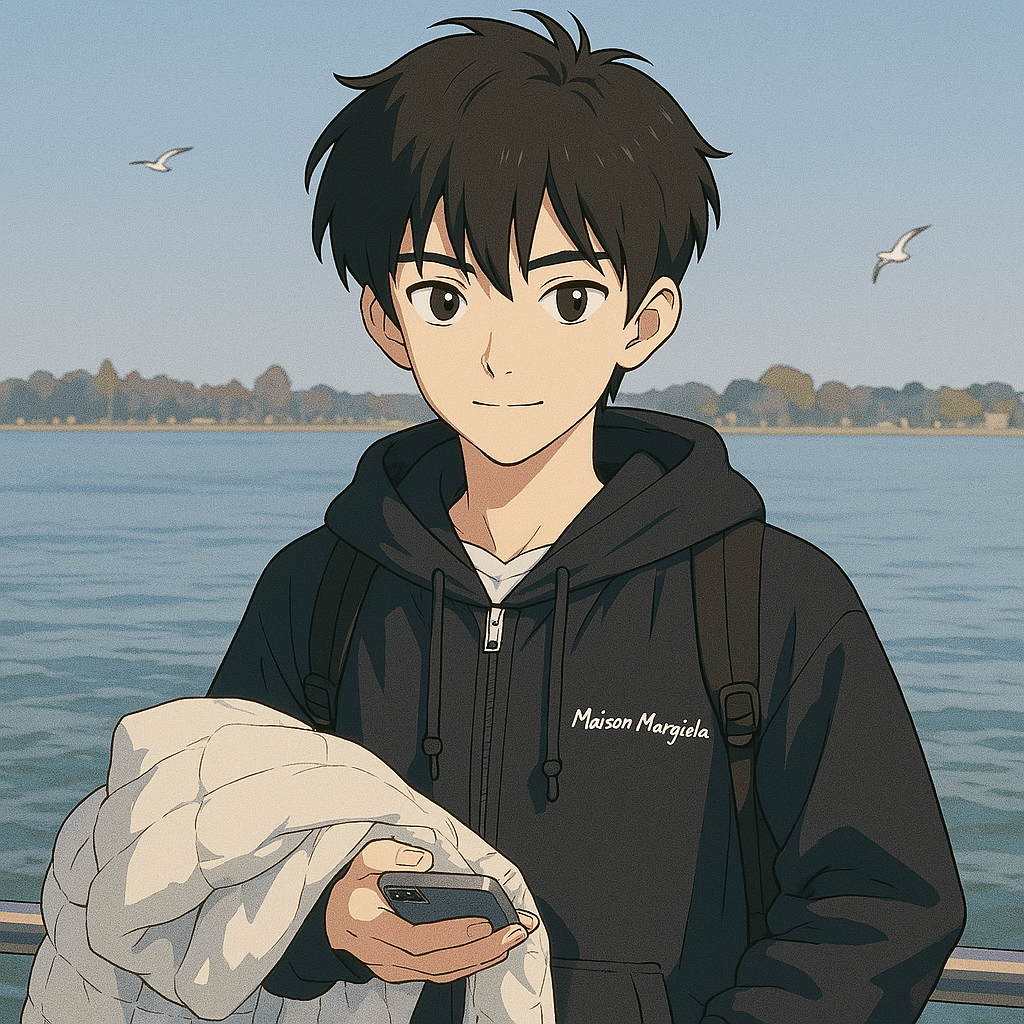
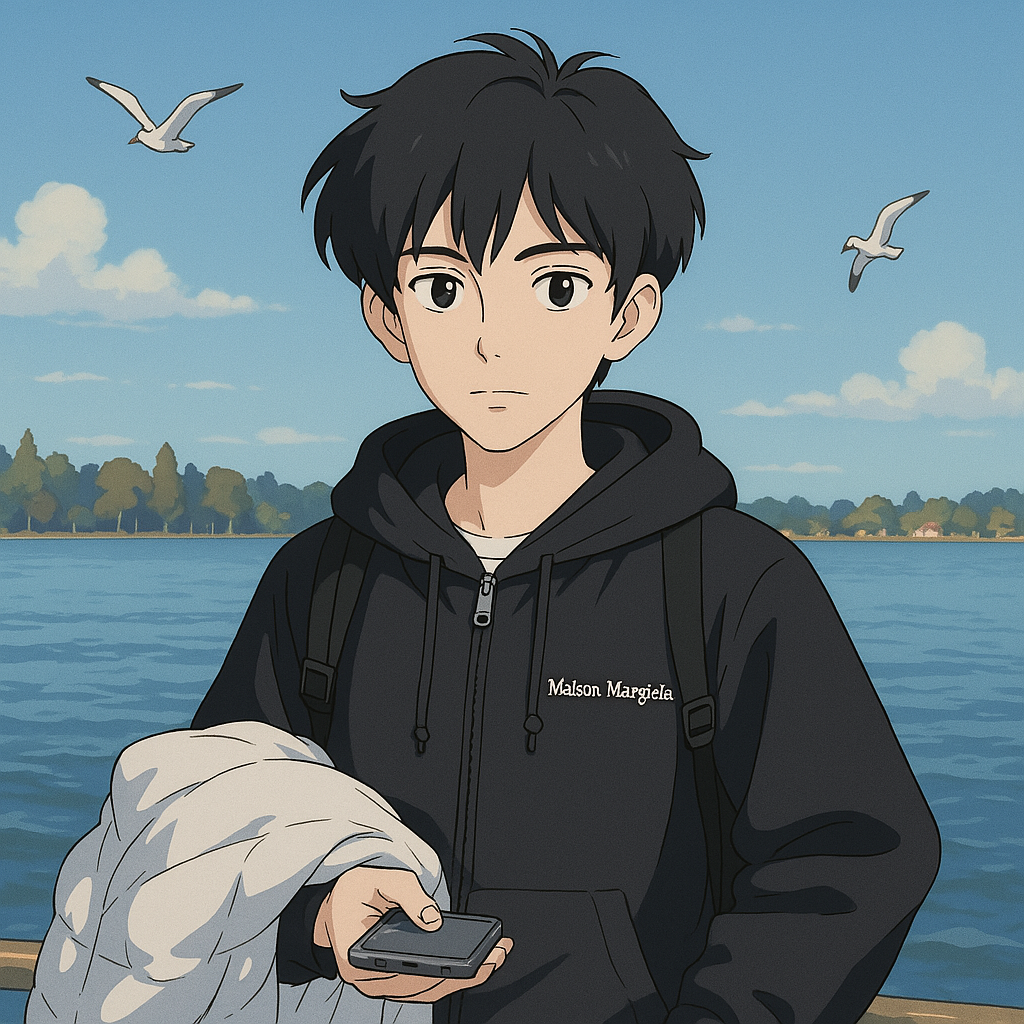
I’m a Ph.D. student (2024–) in Computer Science at UC San Diego 🔱, advised by Prof. Julian McAuley. Prior to this, I completed my Master’s degree at UC San Diego 🎓, working with Julian McAuley, Zhiting Hu, and collaborating with Muhao Chen from UC Davis.
Previously, I interned at NVIDIA 🟩, working with Gaoyan Xie. This summer, I’m joining AWS AI Labs☁️ as an applied scientist intern on the Amazon Q Developer team ⚙️.
I build, train, and evaluate Large Language Models (LLMs) 🧠 — and try to make them smarter 💡.
News
Oct 10, 2024 | 🤖 We pre-release Decentralized Arena for automated, scalable, and transparent LLM evaluation. |
---|---|
Sep 20, 2024 | 🎉 DRPO is accepted to the main conference of EMNLP 2024! |
Jul 10, 2024 | 🎉 LLM Reasoners is accepted to COLM 2024! |
Feb 28, 2024 | 💫 We release StarCoder 2, a family of open LLMs for code. |
Jan 16, 2024 | 🎉 RepoBench gets accepted to ICLR 2024! |
Selected Publications [view all]
2025
- CVPRSymbolic Representation for Any-to-Any Generative TasksJiaqi Chen, Xiaoye Zhu, Yue Wang, Tianyang Liu, Xinhui Chen, Ying Chen, Chak Tou Leong, Yifei Ke, Joseph Liu, Yiwen Yuan, Julian McAuley, and Li-jia LiCVPR, 2025
We propose a symbolic generative task description language and inference engine, capable of representing arbitrary multimodal tasks as symbolic flows. The inference engine maps natural language instructions to symbolic flow, eliminating the need for task-specific training. Conventional generative models rely heavily on large-scale training and implicit neural representation to learn cross-modal mappings, which demands extensive computational resources and restricts expandability. In this paper, we propose an explicit symbolic task descriptive language, comprising three types of primitives: functions, parameters, and topological logic. Using a pre-trained language model to infer symbolic workflows in a training-free manner, our framework successfully performs over 12 multimodal generative tasks based on user instructions, demonstrating enhanced efficiency and flexibility. Extensive experiments demonstrate that our approach can generate multimodal content competitive with, and often surpassing, that of previous state-of-the-art unified models, while offering robust interruptibility and editability. We believe that symbolic task representations are capable of cost-effectively expanding the boundaries of generative AI capabilities.
@article{chen2025symbolic, title = {Symbolic Representation for Any-to-Any Generative Tasks}, author = {Chen, Jiaqi and Zhu, Xiaoye and Wang, Yue and Liu, Tianyang and Chen, Xinhui and Chen, Ying and Leong, Chak Tou and Ke, Yifei and Liu, Joseph and Yuan, Yiwen and McAuley, Julian and Li, Li-jia}, journal = {CVPR}, year = {2025}, }
- preprintDayu Yang*, Tianyang Liu*, Daoan Zhang*, Antoine Simoulin, Xiaoyi Liu, Yuwei Cao, Zhaopu Teng, Xin Qian, Grey Yang, Jiebo Luo, and Julian McAuleyarXiv preprint, 2025
In large language models (LLMs), code and reasoning reinforce each other: code offers an abstract, modular, and logic-driven structure that supports reasoning, while reasoning translates high-level goals into smaller, executable steps that drive more advanced code intelligence. In this study, we examine how code serves as a structured medium for enhancing reasoning: it provides verifiable execution paths, enforces logical decomposition, and enables runtime validation. We also explore how improvements in reasoning have transformed code intelligence from basic completion to advanced capabilities, enabling models to address complex software engineering tasks through planning and debugging. Finally, we identify key challenges and propose future research directions to strengthen this synergy, ultimately improving LLM’s performance in both areas.
@article{yang2025codethinkthinkcode, title = {Code to Think, Think to Code: A Survey on Code-Enhanced Reasoning and Reasoning-Driven Code Intelligence in LLMs}, author = {Yang*, Dayu and Liu*, Tianyang and Zhang*, Daoan and Simoulin, Antoine and Liu, Xiaoyi and Cao, Yuwei and Teng, Zhaopu and Qian, Xin and Yang, Grey and Luo, Jiebo and McAuley, Julian}, journal = {arXiv preprint}, year = {2025}, url = {https://arxiv.org/abs/2502.19411}, }
- AAAIJiaqi Chen*, Xiaoye Zhu*, Tianyang Liu*, Ying Chen, Xinhui Chen, Yiwen Yuan, Chak Tou Leong, Zuchao Li, Long Tang, Lei Zhang, Chenyu Yan, Guanghao Mei, and 2 more authorsAAAI, 2025Oral Presentation
Large Language Models (LLMs) have revolutionized text generation, making detecting machine-generated text increasingly challenging. Although past methods have achieved good performance on detecting pure machine-generated text, those detectors have poor performance on distinguishing machine-revised text (rewriting, expansion, and polishing), which can have only minor changes from its original human prompt. As the content of text may originate from human prompts, detecting machine-revised text often involves identifying distinctive machine styles, e.g., worded favored by LLMs. However, existing methods struggle to detect machine-style phrasing hidden within the content contributed by humans. We propose the "Imitate Before Detect" (ImBD) approach, which first imitates the machine-style token distribution, and then compares the distribution of the text to be tested with the machine-style distribution to determine whether the text has been machine-revised. To this end, we introduce style preference optimization (SPO), which aligns a scoring LLM model to the preference of text styles generated by machines. The aligned scoring model is then used to calculate the style-conditional probability curvature (Style-CPC), quantifying the log probability difference between the original and conditionally sampled texts for effective detection. We conduct extensive comparisons across various scenarios, encompassing text revisions by six LLMs, four distinct text domains, and three machine revision types. Compared to existing state-of-the-art methods, our method yields a 13% increase in AUC for detecting text revised by open-source LLMs, and improves performance by 5% and 19% for detecting GPT-3.5 and GPT-4o revised text, respectively. Notably, our method surpasses the commercially trained GPT-Zero with just 1,000 samples and five minutes of SPO, demonstrating its efficiency and effectiveness.
@article{chen2025imitate, title = {Imitate Before Detect: Aligning Machine Stylistic Preference for Machine-Revised Text Detection}, author = {Chen*, Jiaqi and Zhu*, Xiaoye and Liu*, Tianyang and Chen, Ying and Chen, Xinhui and Yuan, Yiwen and Leong, Chak Tou and Li, Zuchao and Tang, Long and Zhang, Lei and Yan, Chenyu and Mei, Guanghao and Zhang, Jie and Zhang, Lefei}, journal = {AAAI}, year = {2025}, customize = {[demo] https://huggingface.co/spaces/machine-text-detection/ImBD}, url = {https://arxiv.org/abs/2412.10432}, note = {<strong style="color:#cc3333">Oral Presentation</strong>}, }
- pre-releaseYanbin Yin, Zhen Wang, Kun Zhou, Xiangdong Zhang, Shibo Hao, Yi Gu, Jieyuan Liu, Somanshu Singla, Tianyang Liu, Eric P. Xing, Zhengzhong Liu, Haojian Jin, and 1 more authorPre-release, 2025Final version will be released soon.
We release Decentralized Arena that automates and scales “Chatbot Arena” for LLM evaluation across various fine-grained dimensions (e.g., math – algebra, geometry, probability; logical reasoning, social reasoning, biology, chemistry, …). The evaluation is decentralized and democratic, with all LLMs participating in evaluating others. It achieves a 95% correlation with Chatbot Arena’s overall rankings, while being fully transparent and reproducible.
@article{yin2025decentralized, title = {Decentralized Arena via Collective LLM Intelligence: Building Automated, Robust, and Transparent LLM Evaluation for Numerous Dimensions}, author = {Yin, Yanbin and Wang, Zhen and Zhou, Kun and Zhang, Xiangdong and Hao, Shibo and Gu, Yi and Liu, Jieyuan and Singla, Somanshu and Liu, Tianyang and Xing, Eric P. and Liu, Zhengzhong and Jin, Haojian and Hu, Zhiting}, year = {2025}, journal = {Pre-release}, url = {https://de-arena.maitrix.org/}, note = {Final version will be released soon.}, }
2024
- EMNLP (main)EMNLP, 2024
Aligning Large Language Models (LLMs) traditionally relies on costly training processes like supervised fine-tuning (SFT) and reinforcement learning from human feedback (RLHF). To enable alignment without these expensive tuning and annotation, we present a new tuning-free approach for self-alignment called Dynamic Rewarding with Prompt Optimization (DRPO). Our approach enables self-alignment through a search-based prompt optimization framework, allowing the model to self-improve and generate optimized prompts without additional training or human supervision. The core of DRPO leverages a dynamic rewarding mechanism to identify and rectify model-specific alignment weaknesses, enabling LLMs to adapt quickly to various alignment challenges. Empirical evaluations on eight recent LLMs, including both open- and closed-source, reveal that DRPO significantly enhances alignment performance, enabling base models to outperform their SFT/RLHF-tuned counterparts. Moreover, DRPO’s automatically optimized prompts surpass those curated by human experts, demonstrating its superior alignment capabilities. Our findings envision a highly cost-effective and adaptable solution for future alignment research to be further explored.
@article{singla2024dynamic, title = {Dynamic Rewarding with Prompt Optimization Enables Tuning-free Self-Alignment of Language Models}, author = {Singla*, Somanshu and Wang*, Zhen and Liu, Tianyang and Ashfaq, Abdullah and Hu, Zhiting and Xing, Eric P.}, journal = {EMNLP}, year = {2024}, }
- COLMShibo Hao, Yi Gu, Haotian Luo, Tianyang Liu, Xiyan Shao, Xinyuan Wang, Shuhua Xie, Haodi Ma, Adithya Samavedhi, Qiyue Gao, Zhen Wang, and Zhiting HuCOLM, 2024Also to appear at Large Language Model (LLM) Agents workshop at ICLR 2024
Generating accurate step-by-step reasoning is essential for Large Language Models (LLMs) to address complex problems and enhance robustness and interpretability. Despite the flux of research on developing advanced reasoning approaches, systematically analyzing the diverse LLMs and reasoning strategies in generating reasoning chains remains a significant challenge. The difficulties stem from the lack of two key elements: (1) an automatic method for evaluating the generated reasoning chains on different tasks, and (2) a unified formalism and implementation of the diverse reasoning approaches for systematic comparison. This paper aims to close the gap: (1) We introduce AutoRace for fully automated reasoning chain evaluation. Existing metrics rely on expensive human annotations or pre-defined LLM prompts not adaptable to different tasks. In contrast, AutoRace automatically creates detailed evaluation criteria tailored for each task, and uses GPT-4 for accurate evaluation following the criteria. (2) We develop LLM Reasoners, a library for standardized modular implementation of existing and new reasoning algorithms, under a unified formulation of the search, reward, and world model components. With the new evaluation and library, (3) we conduct extensive study of different reasoning approaches (e.g., CoT, ToT, RAP). The analysis reveals interesting findings about different factors contributing to reasoning, including the reward-guidance, breadth-vs-depth in search, world model, and prompt formats, etc.
@article{hao2024llm, title = {LLM Reasoners: New Evaluation, Library, and Analysis of Step-by-Step Reasoning with Large Language Models}, author = {Hao, Shibo and Gu, Yi and Luo, Haotian and Liu, Tianyang and Shao, Xiyan and Wang, Xinyuan and Xie, Shuhua and Ma, Haodi and Samavedhi, Adithya and Gao, Qiyue and Wang, Zhen and Hu, Zhiting}, journal = {COLM}, booktitle = {Conference on Language Modeling}, note = {Also to appear at Large Language Model (LLM) Agents workshop at ICLR 2024}, year = {2024}, }
- preprintAnton Lozhkov, Raymond Li, Loubna Ben Allal, Federico Cassano, Joel Lamy-Poirier, Nouamane Tazi, Ao Tang, Dmytro Pykhtar, Jiawei Liu, Yuxiang Wei, Tianyang Liu, Max Tian, and 54 more authorsarXiv preprint, 2024
The BigCode project, an open-scientific collaboration focused on the responsible development of Large Language Models for Code (Code LLMs), introduces StarCoder2. In partnership with Software Heritage (SWH), we build The Stack v2 on top of the digital commons of their source code archive. Alongside the SWH repositories spanning 619 programming languages, we carefully select other high-quality data sources, such as GitHub pull requests, Kaggle notebooks, and code documentation. This results in a training set that is 4x larger than the first StarCoder dataset. We train StarCoder2 models with 3B, 7B, and 15B parameters on 3.3 to 4.3 trillion tokens and thoroughly evaluate them on a comprehensive set of Code LLM benchmarks. We find that our small model, StarCoder2-3B, outperforms other Code LLMs of similar size on most benchmarks, and also outperforms StarCoderBase-15B. Our large model, StarCoder2- 15B, significantly outperforms other models of comparable size. In addition, it matches or outperforms CodeLlama-34B, a model more than twice its size. Although DeepSeekCoder- 33B is the best-performing model at code completion for high-resource languages, we find that StarCoder2-15B outperforms it on math and code reasoning benchmarks, as well as several low-resource languages. We make the model weights available under an OpenRAIL license and ensure full transparency regarding the training data by releasing the SoftWare Heritage persistent IDentifiers (SWHIDs) of the source code data.
@article{starcoder2, title = {StarCoder 2 and The Stack v2: The Next Generation}, author = {Lozhkov, Anton and Li, Raymond and Allal, Loubna Ben and Cassano, Federico and Lamy-Poirier, Joel and Tazi, Nouamane and Tang, Ao and Pykhtar, Dmytro and Liu, Jiawei and Wei, Yuxiang and Liu, Tianyang and Tian, Max and Kocetkov, Denis and Zucker, Arthur and Belkada, Younes and Wang, Zijian and Liu, Qian and Abulkhanov, Dmitry and Paul, Indraneil and Li, Zhuang and Li, Wen-Ding and Risdal, Megan and Li, Jia and Zhu, Jian and Zhuo, Terry Yue and Zheltonozhskii, Evgenii and Dade, Nii Osae Osae and Yu, Wenhao and Krauß, Lucas and Jain, Naman and Su, Yixuan and He, Xuanli and Dey, Manan and Abati, Edoardo and Chai, Yekun and Muennighoff, Niklas and Tang, Xiangru and Oblokulov, Muhtasham and Akiki, Christopher and Marone, Marc and Mou, Chenghao and Mishra, Mayank and Gu, Alex and Hui, Binyuan and Dao, Tri and Zebaze, Armel and Dehaene, Olivier and Patry, Nicolas and Xu, Canwen and McAuley, Julian and Hu, Han and Scholak, Torsten and Paquet, Sebastien and Robinson, Jennifer and Anderson, Carolyn Jane and Chapados, Nicolas and Patwary, Mostofa and Tajbakhsh, Nima and Jernite, Yacine and Ferrandis, Carlos Muñoz and Zhang, Lingming and Hughes, Sean and Wolf, Thomas and Guha, Arjun and von Werra, Leandro and de Vries, Harm}, journal = {arXiv preprint}, year = {2024}, }
- NAACLTianyang Liu, Fei Wang, and Muhao ChenNAACL, 2024
Large Language Models (LLMs) have shown to be capable of various tasks, yet their capability in interpreting and reasoning over tabular data remains an underexplored area. In this context, this study investigates from three core perspectives: the robustness of LLMs to structural perturbations in tables, the comparative analysis of textual and symbolic reasoning on tables, and the potential of boosting model performance through the aggregation of multiple reasoning pathways. We discover that structural variance of tables presenting the same content reveals a notable performance decline, particularly in symbolic reasoning tasks. This prompts the proposal of a method for table structure normalization. Moreover, textual reasoning slightly edges out symbolic reasoning, and a detailed error analysis reveals that each exhibits different strengths depending on the specific tasks. Notably, the aggregation of textual and symbolic reasoning pathways, bolstered by a mix self-consistency mechanism, resulted in achieving SOTA performance, with an accuracy of 73.6% on WIKITABLEQUESTIONS, representing a substantial advancement over previous existing table processing paradigms of LLMs.
@article{liu2023rethinking, title = {Rethinking Tabular Data Understanding of Large Language Models}, author = {Liu, Tianyang and Wang, Fei and Chen, Muhao}, journal = {NAACL}, booktitle = {Annual Conference of the North American Chapter of the Association for Computational Linguistics}, year = {2024}, }
- ICLRTianyang Liu, Canwen Xu, and Julian McAuleyICLR, 2024
Large Language Models (LLMs) have greatly advanced code auto-completion systems, with a potential for substantial productivity enhancements for developers. However, current benchmarks mainly focus on single-file tasks, leaving an assessment gap for more complex, real-world, multi-file programming scenarios. To fill this gap, we introduce RepoBench, a new benchmark specifically designed for evaluating repository-level code auto-completion systems. RepoBench consists of three interconnected evaluation tasks: RepoBench-R (Retrieval), RepoBench-C (Code Completion), and RepoBench-P (Pipeline). Each task respectively measures the system’s ability to retrieve the most relevant code snippets from other files as cross-file context, predict the next line of code with cross-file and in-file context, and handle complex tasks that require a combination of both retrieval and next-line prediction. RepoBench aims to facilitate a more complete comparison of performance and encouraging continuous improvement in auto-completion systems. RepoBench is publicly available at https://github.com/leolty/RepoBench
@article{liu2023repobench, author = {Liu, Tianyang and Xu, Canwen and McAuley, Julian}, title = {RepoBench: Benchmarking Repository-Level Code Auto-Completion Systems}, journal = {ICLR}, booktitle = {The Twelfth International Conference on Learning Representations}, year = {2024}, }
2023
- NeurIPSNeurIPS, 2023Oral (67 out of 12345 submissions), Best Paper Award at SoCal NLP 2023
Augmenting large language models (LLMs) with external tools has emerged as a promising approach to solving complex problems. However, traditional methods, which finetune LLMs with tool demonstration data, can be both costly and restricted to a predefined set of tools. Recent in-context learning paradigm alleviates these issues, but the limited context length only allows for a few shots of demonstrations, leading to suboptimal understandings of the tools. Moreover, when there are numerous tools to choose from, in-context learning could completely fail to work. In this paper, we propose an alternative approach, ToolkenGPT, which combines the benefits of both sides. Our approach represents each tool as a ken (i.e., toolken) and learns an embedding for it, enabling tool calls in the same way as generating a regular word token. Once a toolken is triggered, the LLM is prompted to complete arguments for the tool to execute. ToolkenGPT offers the flexibility to plug in an arbitrary number of tools by expanding the set of toolkens on the fly. In addition, it improves tool use by allowing extensive demonstration data for learning the toolken embeddings. In diverse domains, including numerical reasoning, knowledge-based question answering, and embodied plan generation, our approach effectively augments LLMs with tools and substantially outperforms various latest baselines. ToolkenGPT demonstrates the promising ability to use relevant tools from a large tool set in complex scenarios.
@article{hao2023toolkengpt, title = {ToolkenGPT: Augmenting Frozen Language Models with Massive Tools via Tool Embeddings}, author = {Hao, Shibo and Liu, Tianyang and Wang, Zhen and Hu, Zhiting}, journal = {NeurIPS}, note = {<strong style="color:#cc3333">Oral (67 out of 12345 submissions), Best Paper Award at SoCal NLP 2023</strong>}, year = {2023}, }
Services
Invited Reviewer
- AAAI
- AISTATS
- ACL ARR (Feb)
- COLM
- ICLR
- ICML
- ACL ARR (Feb, Apr, Jun, Aug, Oct, Dec)
- COLM
- ICLR
- ICML
- NeurIPS
- ACL ARR (Dec)
- NLPCC